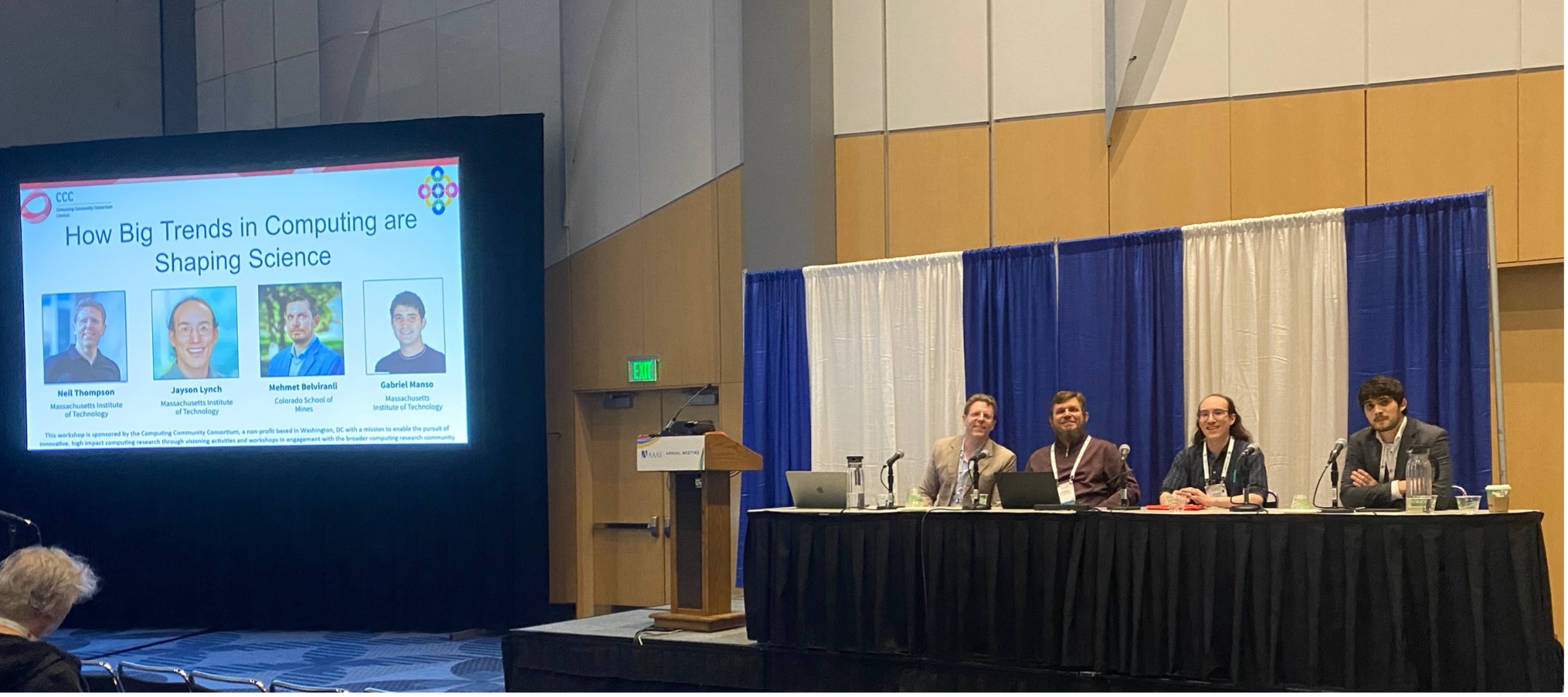
CCC supported three scientific periods at this 12 months’s AAAS Annual Convention, and in case you weren’t in a position to attend in individual, we’re recapping every session. This week, we’ll summarize the highlights of the session, “How Big Trends in Computing are Shaping Science.” In Half 1, we’ll hear from Dr. Neil Thompson, from the Massachusetts Institute of Expertise, who will clarify the computing tendencies shaping the way forward for science, and why they are going to affect almost all areas of scientific discovery.
CCC’s third AAAS panel of the 2024 annual assembly befell on Saturday, February seventeenth, on the final day of the convention. The panel, comprised Jayson Lynch (Massachusetts Institute of Expertise), Gabriel Manso (Massachusetts Institute of Expertise), and Mehmet Belviranli (Colorado Faculty of Mines), and was moderated by Neil Thompson (Massachusetts Institute of Expertise).
Neil Thompson, director of the FutureTech mission at MIT, kicked off the panel by giving background on the precise tendencies in computing that the panel supposed to deal with, these being the top of Moore’s Legislation and the rise of Synthetic Intelligence. “All through this panel”, mentioned Dr. Thompson, “we’ll focus on how these tendencies are shaping not solely computing however a lot of scientific discovery.” Climate prediction, for instance, is one thing computer systems have been used on for a really very long time. Again within the Fifties, researchers started to make use of computer systems for climate prediction, and the graph under shows the ability of the techniques NOAA used to make these predictions over time. The Y axis exhibits the computing energy, and every leap on the graph is an element of 100. “Over this era from 1955 to 2019”, mentioned Dr. Thompson, “we’ve got had about one trillion-fold enhance within the quantity of computing getting used for these techniques. Over this era, we’ve got observed not solely an unbelievable progress within the energy of those techniques but additionally an unlimited enhance within the accuracy of those predictions.”
The graph under shows the error price of those predictions in levels Fahrenheit over a rise in computing energy over the identical time scale (1955 – 2019). “We see an extremely robust correlation between the quantity of computing energy and the way correct the prediction is”, defined Dr. Thompson. “For instance, for predictions three days out, 94% of the variance in prediction high quality is defined by the will increase in computing energy.”
Researchers are usually not simply utilizing extra computing energy; the supply of elevated computing energy permits scientists to develop higher algorithms that, consequently, can harness this energy extra effectively and get extra out of those highly effective fashions. For the previous 40 to 50 years, we’ve got been in a position to depend on extra computing energy as a result of Moore’s Legislation had not but reached its limits, and computing energy has continued to develop regularly with out vital will increase in price. Nonetheless, with Moore’s Legislation coming to an finish, scientists might now not depend on common will increase in computing energy with low related prices as they did earlier than. The graph under exhibits this development slowing. The Y axis on the graph exhibits the relative efficiency in comparison with a system in 1985, and every dot represents a single computing system. The inexperienced dots present how briskly these computer systems run when it comes to the clock frequency. “We noticed exponential enhancements for many years till it plateaued in 2005 on the finish of Dennard scaling when chips might now not run sooner. Scientists started to make use of different strategies to extend efficiency, equivalent to utilizing multi-core processors”, mentioned Dr. Thompson. The black dots present this shift, throughout which era researchers continued to see exponential enhancements. Serialized computing, as proven within the grey line, is unable to benefit from parallel computing, and we will see that progress slowed manner down after 2005. “With elevated parallelism, we hope to proceed leveraging the rising computing energy that we’re used to, a development that additionally accounts for the rising manufacturing of extra specialised chips”, defined Dr. Thompson.
Dr. Thompson then turned the viewers’s consideration to deep studying. “On the subject of prices, what’s going on in deep studying appears very totally different from different kinds of science. The determine under shows the share of analysis prices for various analysis initiatives which can be spent on capital, such because the {hardware} and tools essential to conduct these sorts of analysis. In most areas of analysis, the amount of cash allotted in the direction of capital is constrained to twenty% or much less. Nonetheless, the analysis being achieved in synthetic intelligence often requires a couple of third of the funds to be spent on capital.”
Provided that AI analysis could be very costly and capital-intensive, we will see very totally different performances in numerous duties. Predicting protein folding, for instance, has seen unbelievable progress since we started utilizing AI to foretell these patterns. The graph under shows totally different analysis teams who’re finding out protein folding utilizing AI. “Alphafold 1 and a couple of, developed by DeepMind, have achieved exceptional success and are positioned prominently on this continuum. Their considerably greater accuracy charges are largely attributed to their use of techniques that harness computing energy on a scale a lot bigger than these employed by the teams to the left. This means that entry to substantial computing assets will play a vital function in advancing scientific progress.”
Thanks a lot for studying, and keep tuned tomorrow for our abstract of Gabriel Manso’s presentation on The Computational Limits of Deep Studying.