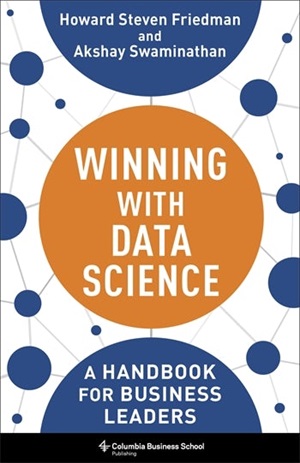
About 90% of companies say they plan to extend their investments in information, analytics and AI, however many companies are struggling to realize worth from information science investments. These failures result in a waste of cash, time and alternative for the corporate.
Knowledge science efforts can fail for 2 primary causes: business-related causes and data-related causes. On this first of two articles, tailored from our ebook Profitable with Knowledge Science, we’ll focus on a number of the most typical business-related causes for these failures and what you are able to do to keep away from these pitfalls. Our follow-up article will discover a number of the data-related points.
Earlier than detailing a number of the key business-issues, you will need to emphasize that enterprise prospects (in any other case generally known as stakeholders or collaborators) play a vital function within the success (or failure) of data-related tasks. They’re the info science workforce’s prospects and they also must be actively engaged in all elements of the mission. Good prospects talk their wants, make investments time, ask the suitable questions, problem assumptions, and study the language.
Some necessary business-related errors in information tasks embrace:
- Specializing in sizzling tech as a substitute of issues: It’s simple to get distracted by the subsequent huge factor in tech, however information tasks ought to remedy a essential enterprise downside, like rising income or decreasing bills. Beginning with the answer, whether or not that be Generative AI or deep studying, after which searching for the suitable downside is a recipe for bother.
- Not defining success: The information science groups must know the targets of the mission and the way success is measured. Being clear about 5 dimensions are necessary: the anticipated use of the product, time anticipated to construct, value of the mission, the standard required and the satisfaction of the stakeholders. Your definition of success must be achievable so open communication and suggestions from the info science workforce is essential
- Not being engaged: Merely hiring an information science workforce will not be sufficient. The information science buyer has to remain engaged within the course of and take part in mission administration conferences to grasp the important thing milestones, challenges and alternatives. Being an engaged buyer will go an extended strategy to guaranteeing success.
- Not asking questions: Communication is a essential a part of any information science mission. The client ought to at all times ask questions that span the vary of mission administration, product choices, modeling, and particular suggestions made by the info science workforce. Most significantly, if the solutions aren’t clear, then the shopper ought to proceed to ask questions – information scientists ought to have the ability to clarify what they’re doing and why in a language that’s readily understood by non-specialists.
- Accepting assumptions: The enterprise buyer ought to problem assumptions made by the info science workforce. In any case, the enterprise buyer reveals up with material information and expertise. They’ve a deep understanding of the enterprise and will perceive why one definition of an consequence variable is healthier than one other or can clarify what’s the minimal mannequin efficiency wanted in the actual world.
- Not Studying the Language: Enterprise prospects ought to study a number of the fundamental phrases of knowledge science. This doesn’t imply they should code in Python, use Github or carry out head-to-head exams of Generative AI fashions. It means they need to perceive key phrases associated to options, consequence variables, efficiency metrics and fundamental statistics.
- Ignoring Ethics considerations: Failing to completely handle ethics considerations can lead to potential biases, equity points, and privateness considerations that may injury belief and fame. The very last thing you need is to have your organization seem on the Wall Road journal entrance web page for example of algorithm bias or AI ethics failures. Failing to concentrate to ethics considerations can create issues for the enterprise proprietor, the corporate itself and people the corporate’s prospects.
The errors above all stem from failures in planning, preparation and communication. The excellent news is that these errors are avoidable. Earlier than initiating an information science mission, we suggest the enterprise buyer takes the time to evaluation this checklist above and determine what steps they are going to take to keep away from the pitfalls and enhance the possibilities of success.
Tailored from Winning with Data Science by Howard Steven Friedman and Akshay Swaminathan, printed by Columbia Enterprise College Publishing. Copyright (c) 2024 Howard Steven Friedman and Akshay Swaminathan. Utilized by association with the Writer. All rights reserved.
Concerning the Authors
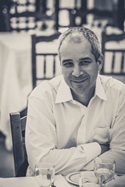
Howard Steven Friedman is an information scientist, well being economist, and author with many years of expertise main information modeling groups within the non-public sector, public sector, and academia. He’s an adjunct professor, educating information science, statistics, and program analysis, at Columbia College, and has authored/co-authored over 100 scientific articles and ebook chapters in areas of utilized statistics, well being economics and politics. His earlier books embrace Final Worth and Measure of a Nation, which Jared Diamond known as the very best ebook of 2012.
.
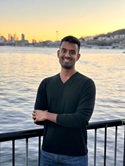
Akshay Swaminathan is an information scientist who works on strengthening well being programs. He has greater than forty peer-reviewed publications, and his work has been featured within the New York Occasions and STAT. Beforehand at Flatiron Well being, he presently leads the info science workforce at Cerebral and is a Knight-Hennessy scholar at Stanford College College of Drugs.
.
Join the free insideBIGDATA newsletter.
Be part of us on Twitter: https://twitter.com/InsideBigData1
Be part of us on LinkedIn: https://www.linkedin.com/company/insidebigdata/
Be part of us on Fb: https://www.facebook.com/insideBIGDATANOW