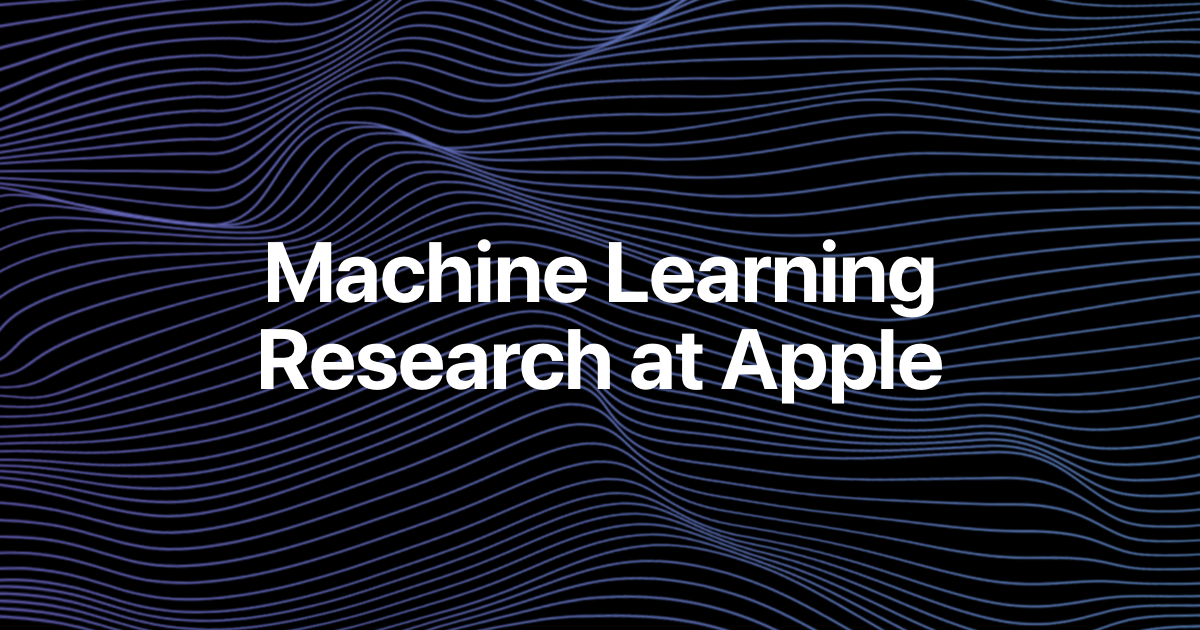
This paper introduces a novel generative modeling framework grounded in section area dynamics, taking inspiration from the rules underlying Critically Damped Langevin Dynamics (CLD). Leveraging insights from stochastic optimum management, we assemble a positive path measure within the section area that proves extremely advantageous for generative sampling. A particular characteristic of our method is the early-stage knowledge prediction functionality inside the context of propagating producing Odd Differential Equations (ODEs) or Stochastic Differential Equations (SDEs) processes. This early prediction, enabled by the mannequin’s distinctive structural traits, units the stage for extra environment friendly knowledge technology, leveraging further velocity data alongside the trajectory. This innovation has spurred the exploration of a novel avenue for mitigating sampling complexity by transitioning straight from noisy knowledge to genuine pictures. Our mannequin yields comparable ends in picture technology and notably outperforms baseline strategies, notably when confronted with a restricted Variety of Perform Evaluations (NFE). Moreover, our method rivals the efficiency of diffusion fashions outfitted with environment friendly sampling methods, underscoring its potential within the realm of generative modeling.